Supernormal Neural Networks
- The Natural Philosopher
- Apr 11, 2022
- 2 min read
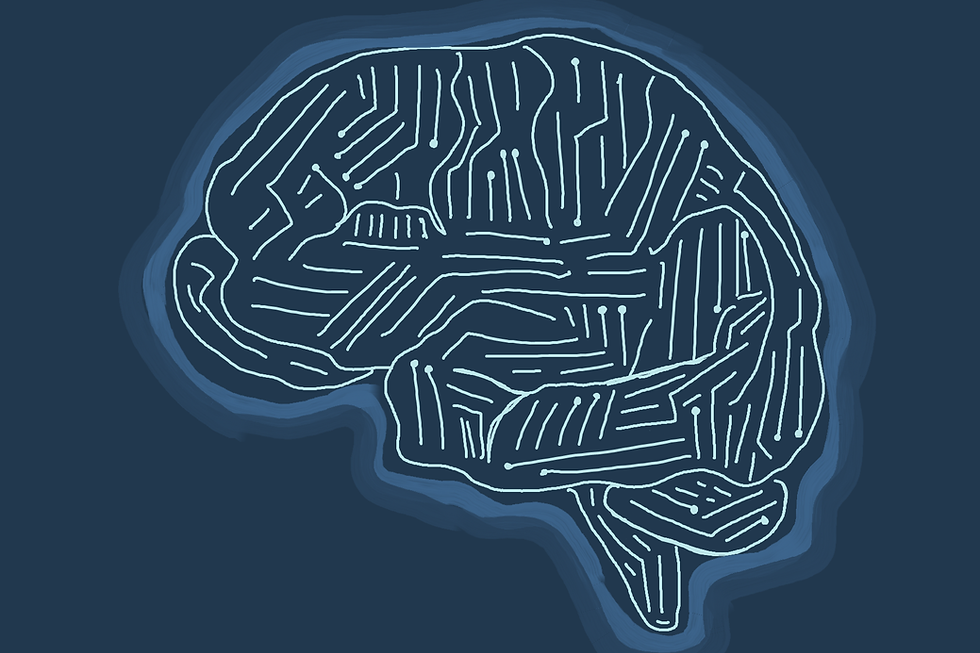
By Conor Doolin
The brain is an extremely complex system that can perform a huge variety of tasks. Deep learning systems use data and algorithms to train artificial intelligence (AI) machines. Artificial intelligence is inspired by the creative arrangements of human consciousness. The main difference between AI and human intelligence is that humans rely on past mistakes or gained knowledge to perform tasks while AI machines depend on complex input data [1].
AI machines shape a future of automated tasks, economic growth, creativity, and innovation. These tasks can translate to financial estimates, automated driving, smart home security, and clinical healthcare decision systems [2]. In the healthcare sector, AI falls into two main categories: supervised and unsupervised [2]. Supervised methods include labeled data, which acts as raw data input for machine learning systems, such as X-ray detection tracers, implantable cardiac device feedback interpretation, and prognosis models of infectious diseases [2]. Unsupervised learning models work to solve more complex problems such as hidden patterns. These patterns could contribute to pharmacogenomics, a branch of genetics concerned with individuals’ genetic attribution to therapeutic drugs. Also, reinforced learning, a deep learning system training method based on validating desired outcomes and/or highlighting undesired ones, could maximize the accuracy of disease prediction and treatment outcomes. Precision health, drug discovery, repurposing, and epidemic outbreak prediction models could become more accurate and precise with the support of deep learning machines [2].
Unfortunately, there are many challenges and alternative perspectives that prevent forward progress in technological personalized medicine [2]. First, there are computational challenges associated with algorithms capable of improving positive patient health care efficacy. Algorithms cannot encompass suitable predictions for all genetic diversity across human populations, so data and insight need to form continuous evolving arrays of questions [2]. Secondly, the volume becomes an issue with safe, applicable AI healthcare decision systems. The scale of data inputs and outputs presents challenges with clusters of CPUs data sharing between the cloud and performing deep-learning executions instantaneously [2]. Lastly, ethics presents a huge debate as data privacy is defined as a fundamental human right [2]. This protection of privacy must be balanced with the potential ability of health care institutions to improve patient care by communicating data with other practitioners or patients. Moreover, a convergence of medical technologies and a centralized electronic medical record can improve AI deep learning system algorithms ultimately revolutionizing patient care and accessibility to the complexities of data insight. In the end, it may be humanitarian to further develop the theories and algorithms of AI and substitute human tasks with deep human-machine learning integration.
Works Cited:
[1] M. Ergen, “What is Artificial Intelligence? Technical Considerations and Future Perception,” Anatol. J. Cardiol., 2019, doi: 10.14744/AnatolJCardiol.2019.79091.
[2] N. Noorbakhsh-Sabet, R. Zand, Y. Zhang, and V. Abedi, “Artificial Intelligence Transforms the Future of Health Care,” Am. J. Med., vol. 132, no. 7, pp. 795–801, Jul. 2019, doi: 10.1016/j.amjmed.2019.01.017.
เว็บพนันออนไลน์ของเรานั้นได้มีเกมพนันมากมายให้ท่านได้เลือกเล่นมากกว่า 100 เกม ท่านรู้ไหมว่าท่านสามารถที่จะเข้ามาเล่นเกมพนันได้อย่างเต็มรูปแบบได้เลย และแน่นอนว่าทางเว็บแทงบอล ของเรานั้นได้เปิดให้ทุก ๆ ท่านได้เข้ามาสมัครสมาชิกได้ง่ายมาก ๆ เพียงไม่กี่ขั้นตอนเท่านั้นเอง ซึ่งเราอยากให้ทุก ๆ ท่านได้เข้ามาเล่นเกมพนันได้อย่างเต็มรูปแบบได้เลย แบบที่ท่านไม่ต้องกลัวอะไรแม้แต่นิดเดียว
This post talks about Supernormal Neural Networks. The brain is a very sophisticated organ that is capable of carrying out a vast array of jobs. Deep learning systems train artificial intelligence (AI) computers using data and algorithms. The imaginative configurations of human mind serve as a model for artificial intelligence. The primary distinction between human intelligence and artificial intelligence is that while humans rely on learned behavior or previous failures to complete tasks, AI devices require complicated input data. That's why I support your post.Now,I, I am looking for an expert to finish my assignment such as top rated dissertation writing services. Amazing work and good luck in the future.